Wassila Grine
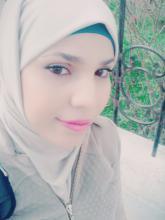
- grine.wassila@univ-guelma.dz
Thesis title
Thesis title (Ar)
Thesis title (Fr)
keywords
keywords (Ar)
keywords (Fr)
Abstract
Nanofluids have recently been considered one of the most popular working fluids in heat transfer and fluid mechanics. A precise estimation of the thermophysical properties of nanofluids is necessary for the study of their heat transfer performance. Thermal conductivity coefficient and viscosity are some of the most important thermophysical properties that directly influence the application of nanofluids.
An increase in the thermal conductivity of nanofluids cannot only be attributed to the greater thermal conductivity of the suspended nanoparticles. But, the improvement comes from a combination of several simultaneous physical factors of varying importance. At this nanometric scale, thermal behavior does not necessarily share the models of larger structures, which requires doing more research to design appropriate models to explain what we observe.
In this context, the main objective of this research work is to develop a simple and precise numerical model capable of modeling the thermal conductivity of metal oxides / water nanofluids, by using an experimental design (Design of Experiments DOE). This model is then tested and analyzed by the ANOVA method in order to determine different factors’ effects of and their interactions. The obtained results show that the volume fraction of the particles has most significant effect in improving nanofluids thermal conductivity compared to the other factors. Unlike the effect of particle size, increasing the volume fraction and temperature of the particles has a positive effect on improving nanofluids thermal conductivity. The R2 and RMSE values are of 0.99 and 0.0074 respectively; indicating that the TCR model provides a good correlation hence fitting perfectly predicted and experimental values. Consequently, the results obtained confirm the capacity of the proposed model of our study to predict nanofluids thermal conductivity with great precision.
The second objective of the present research is to study Artificial Neural Networks (ANNs) application to predict thermophysical properties of (Al2O3/Water) nanofluid. The nanofluid thermal conductivity and its viscosity are thus respectively modeled. The results of this modeling show that the best architecture found for the rate of TCR (knf/kf) and DVR ( ), is of 15 and 20 neurons in the hidden layer respectively. The model of ) provides a good correlation between predicted and experimental values with values of R2 equal to 0.998 and 0.998 for both learning and validation. In addition, the MSE values are of 0.000018 for learning and 0.0000162 for validation. An excellent correlation is also noticed between the predicted and observed response for (), with thus a higher values of R²=0.999 and a lower values of MSE=0.000788 for training and R²=0.999 and SME=0.000678 for validation.
Comparison with various empirical correlations, taking into account the AARD% values of 0.013 % for () and of 0.0019 % for (), confirms that the proposed model has greater performance in the prediction of TCR and DVR of Alumina/Water nanofluids and thus can be considered as a practical tool for the considered task.
Abstract (Ar)
مؤخرًا تعتبر الموائع النانوية واحدة من أكثر سوائل العمل شيوعًا في مجال نقل الحرارة وميكانيكا السوائل. يعد التقدير الدقيق للخصائص الفيزيائية الحرارية للسوائل النانوية ضروريًا لدراسة أداء نقل الحرارة. يعتبر معامل التوصيل الحراري واللزوجة من أهم الخصائص الفيزيائية الحرارية التي تؤثر بشكل مباشر على تطبيق السوائل النانوية.
لا يمكن ان تنسب هذه الزيادة في التوصيل الحراري للسائل النانوي إلى الموصلية الحرارية العالية للجسيمات النانوية المعلقة فقط. أنما التحسين يأتي من مزيج عدة عوامل فيزيائية متزامنة ذات أهمية متفاوتة. في هذا المقياس النانوي ، لا يشترك السلوك الحراري بالضرورة في نماذج ذات الهياكل الأكبر ، الأمر الذي يتطلب إجراء المزيد من الأبحاث لتصميم نماذج أخرى أكثر ملاءمة لشرح ما نلاحظه.
في هذا السياق ، يتمثل الهدف الرئيسي لهذا العمل البحثي في تطوير نموذج رقمي بسيط ودقيق قادر على نمذجة التوصيل الحراري لأكاسيد المعادن / موائع المياه النانوية ، باستخدام تصميم تجريبي (تصميم التجارب (DOE ، بعد ذلك يتم اختبار هذا النموذج وتحليله بواسطة طريقة ANOVA لتحديد تأثيرات العوامل المختلفة وتفاعلاتها. أظهرت النتائج المتحصل عليها أن حجم الجسيمات له تأثير أكبر في تحسين التوصيل الحراري للموائع النانوية مقارنة بالعوامل الأخرى. و على عكس تأثير قطر الجسيمات ، فإن زيادة تركيز الحجم ودرجة حرارة الجزيئات له تأثير إيجابي على تحسين التوصيل الحراري للسوائل النانوية. قيمتا R2 (معامل الانحدار الخطي) 0.99 : و RMSE (جذر متوسط الخطأ التربيعي) : 0.0074 على التوالي وهذا يدل على أن نموذج TCR (نسبة التوصيل الحراري) يوفر علاقة جيدة وأن القيم المتوقعة والتجريبية مناسبة تمامًا. أخيرًا ، تؤكد النتائج التي تم الحصول عليها قدرة النموذج المقترح في دراستنا على التنبؤ بالتوصيل الحراري للسوائل النانوية بدقة كبيرة.
الهدف الثاني هو دراسة تطبيق الشبكات العصبية الاصطناعية (RNA) للتنبؤ بالخصائص الفيزيائية الحرارية للسائل النانوي (أكسيد الألومنيوم/ الماء). تم تصميم الموصلية الحرارية ولزوجة السائل النانوي على التوالي. تظهر نتائج هذه النمذجة أن أفضل بنية تم العثور عليها لمعدل TCR(نسبة التوصيل الحراري( ( ) وDVR (نسبة اللزوجة الديناميكية( ( ) هي 15 و 20 خلية عصبية في الطبقة المخفية على التوالي. يوفر النموذج ( ) ارتباطًا جيدًا بين القيم المتوقعة والتجريبية بقيم (معامل الانحدار الخطي) R2 تساوي 0.998 لكل من التعلم والتحقق، بالإضافة إلى قيم MSE (متوسط الخطأ التربيعي) البالغة 0.000018 للتعلم و 0.0000162 لـلتحقق. كما لوحظ وجود ارتباط ممتاز بين الاستجابة المتوقعة والملاحظة لـ ( ) وبالتالي ، فإن القيم الأعلى لـ R² ═ 0.999 والأقل لـ MSE ═ 0.000788 للتعلم ، كذلك القيم الأعلى لـ R² ═ 0.999 والأقل لـ MSE ═ 0.000678 للتحقق من الصحة.
المقارنة مع الارتباطات التجريبية المختلفة مع الأخذ في الاعتبار قيم AARD%لـ ( )هي 0.0019 % و0.013 % لـ ( ) يؤكد أن النموذج المقترح له أداء متفوق في التنبؤ بـ TCR و DVR للسوائل النانوية (الألومينا/ الماء) ويمكن اعتباره أداة عملية للمهمة المدروسة.
Abstract (Fr)
Les nanofluides ont récemment été considérés comme l'un des fluides de travail les plus populaires en transfert de chaleur et en mécanique des fluides. Une estimation précise des propriétés thermophysiques des nanofluides est nécessaire pour l'étude de leurs performances en transfert de chaleur. Le coefficient de conductivité thermique et la viscosité sont quelques-unes des propriétés thermophysiques les plus importantes qui influencent directement l'application des nanofluides.
Une telle augmentation de la conductivité thermique du nanofluide ne peut pas seulement être imputée à la plus grande conductivité thermique des nanoparticules en suspension. Mais, l'amélioration vient d'une combinaison de plusieurs facteurs physiques simultanés d'importance variable. À cette échelle nanométrique, le comportement thermique ne partage pas forcément les modèles des structures plus grandes, ce qui impose de faire plus de recherche pour en concevoir d'autres mieux adaptés afin d'expliquer ce que l'on observe.
Dans ce contexte, l’objectif principal de ce travail de recherche est de développer un modèle numérique simple et précis capable de modéliser la conductivité thermique des nanofluides d’Oxydes métalliques/Eau, en utilisant un plan d'expérience (Design of Experiments DOE), ensuit ce modèle est testé et analysé par la méthode ANOVA pour déterminer les effets des différents facteurs et leurs interactions. Les résultats obtenus montrent que la fraction volumique des particules présente un effet plus significatif dans l’amélioration de la conductivité thermique des nanofluides par rapport aux autres facteurs. Contrairement à l'effet de la taille des particules, l'augmentation de la fraction volumique et de la température des particules possède un effet positif sur l'amélioration de la conductivité thermique des nanofluides. Les valeurs R2 et RMSE de 0,99 et 0,0074 respectivement, indiquent ainsi que le modèle de TCR fournit une bonne corrélation et que les valeurs prédites et expérimentales s’adaptent parfaitement. Enfin, les résultats obtenus par DOE confirment la capacité du modèle proposé à prédire la conductivité thermique des nanofluides avec une grande précision.
Le second objectif de ce travail est l’étude de l’application des réseaux de neurones artificiels (RNA) pour prédire les propriétés thermophysiques du nanofluide (Al2O3/Eau). La conductivité thermique TCR ( ) et la viscosité DVR ( ) du nanofluide sont respectivement modélisées. Les résultats de cette modélisation montrent que la meilleure architecture trouvée pour le taux de TCR et DVR est de 15 et 20 neurones dans la couche cachée respectivement. Le modèle ( ) fournit une bonne corrélation entre les valeurs prédites et expérimentales avec des valeurs de R2 égales à 0,998 et 0,998 pour l'apprentissage et la validation. De plus, puisque les valeurs de MSE sont de 0,000018 pour l'apprentissage et de 0,0000162 pour la validation pour ( ), donnant ainsi une excellente corrélation entre la réponse prédite et observée ; avec des valeurs plus élevées de R² = 0,999 et de valeurs faibles de MSE = 0,000788 pour l'apprentissage et R² = 0,999 et SME = 0,000678 pour la validation.
La comparaison avec les diverses corrélations empiriques prenant en compte les valeurs AARD de 0.0019 % pour ( ) et de 0.013 % pour ( ) confirme que le modèle proposé à des performances supérieures dans la prédiction du TCR et du DVR des nanofluides Alumine/Eau et peut être considéré comme un outil pratique pour la tâche considérée.
Scientific publications
Grine W, Benhamza MEH. “Modeling the effective thermal conductivity of nanofluids using full factorial design analysis” Journal: Heat Transfer—Asian Res. Online ISSN:1523-1496. Volume 48, Issue 7, Pages: 2930‐2947 November 2019/ https://doi.org/10.1002/htj.21524
Scientific conferences
Communications internationales: 1*” THERMAL CONDUCTIVITY MODELING OF OXIDE-WATER NANOFLUIDS USING “JMP” ", The Third International Conference on “Research to Applications & Markets”, Hammamet, TUNISIA, September 16-18, 2016,http://www.agendas.ovh/third-international-conference-on-research-to-app... 2*” Using Design Of Experiments “DOE” In Modeling Of Effective Thermal Conductivity Of Nanofluids”, the 4th International Conference on Pure and Applied Sciences Nov.23-25, 2017, Gelisim University, Istanbul, Turkey, http://confs.naturalspublishing.com/icpas2017b/ 3* “ Application of Artificial Neural Network (ANN) for the prediction of thermal conductivity and viscosity of alumina water-based nanofluids”, Vth International Conference on Energy, Materials, Applied Energetics and Pollution(ICEMAEP 2019) , 22-24 October 2019, Constantine, Algeria. https://www.scribd.com/document/410910327/1st-Call-for-Papers-icemaep2019 4* “Application of artificial neural network (ANN) for the prediction of nanofluids thermal conductivity” 6è JSFM-CMC 2019, 17-18 November 2019 - Annaba (Algérie), http://www.jsfm-cmc.com/ Communications nationales: 1* First National Day on “Simulation and Nanomaterials”, December 17, 2014, University Mai 8 1945 Guelma, Algeria. http://www.univ-guelma.dz/fr/news-events/simulation-et-nanomat%C3%A9riau... 2* “ Prediction of thermal conductivity of alumina water-based nanofluids using experimental data and artificial neural network” Journée Nationale « Etude des Matériaux: Elaboration et Modélisation », Dec.13, 2018, Guelma, Algeria. http://www.univ-guelma.dz/fr/journ%C3%A9e-nationale-%C2%AB%C3%A9tude-des... 3*“Predicting the effective thermal conductivity of Alumina/water and Alumina/Ethylene glycol nanofluids using artificial neural network” 8èmes Journées de Chimie Ecole Militaire Polytechnique (EMP) Chahid Abderrahmane Taleb Bordj El-Bahri, Algiers, Algeria. March 26-27, 2019. http://www.emp.mdn.dz/events/jch/ 4*“Application of Artificial Neural Network for the prediction of oxide-based nanofluids dynamic viscosity”, 2nd Conference on Informatics and Applied Mathematics IAM'2019, 12-13 June 2019, Guelma, Algeria. http://www.univ-guelma.dz/fr/2nd-conference-informatics-and-applied-math...